Improving AI Accuracy: The Impact of Removing 3 Knowledge Base Article Types
Feb 10, 2025
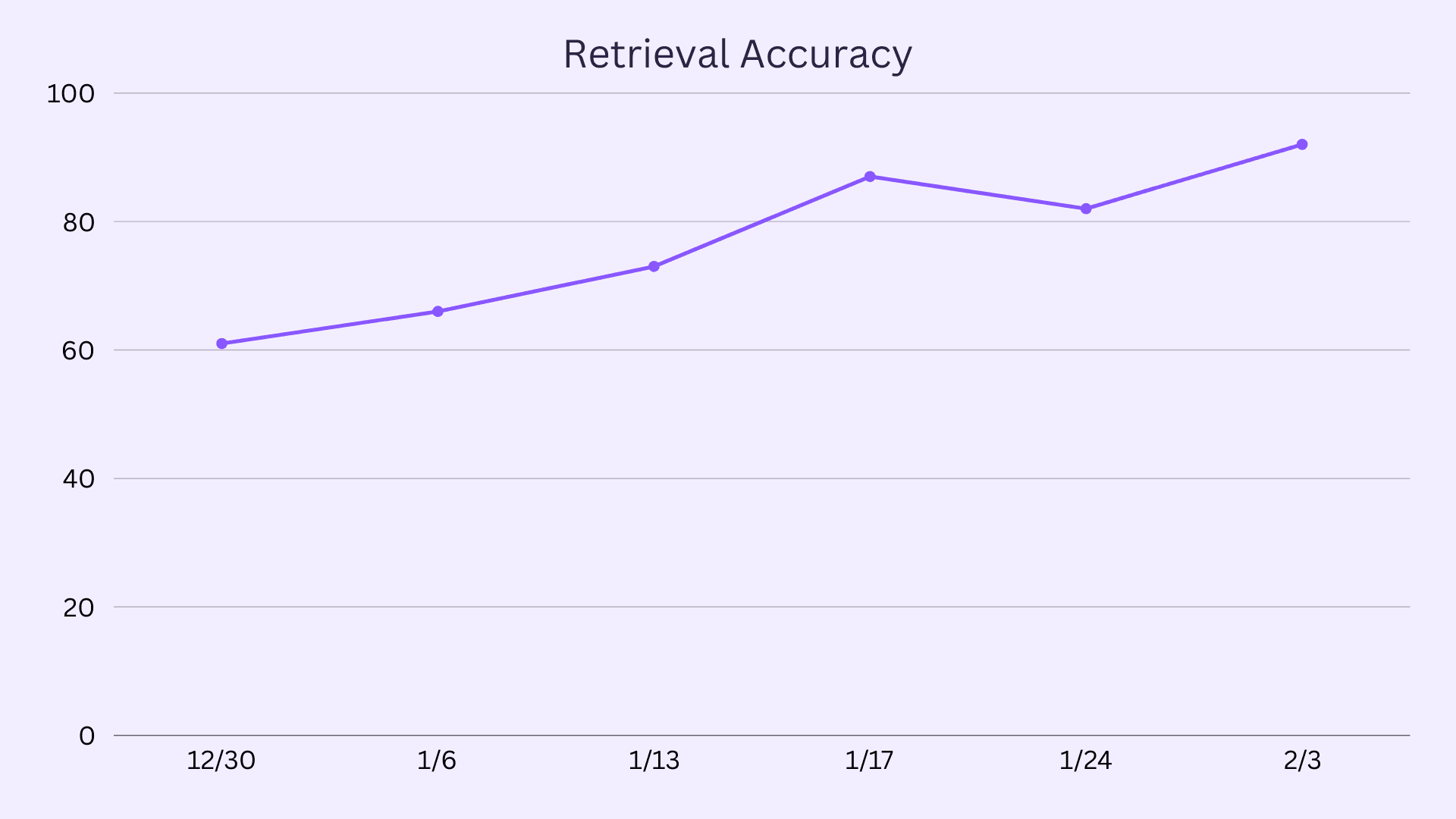
At Stylo, we’re always trying to dogfood our own products - but back in December, we decided to do an Accuracy and Quality review on ourselves, similar to what we do for our customers.
Doing this, we realized our own retrieval system wasn’t performing as well as it should with our own Help Center.
Our RAG-based system had dipped to an honestly terrible 62%, and we were facing the same issue many of our customers do. That is, irrelevant or inaccurate responses slowing down our support and sales teams.
We didn’t want to be a “do as we say, not as we do” kind of company, so we decided to run our beta product, Stylo Scorecard, on our own Help Center.
What we found was that there were three major types of articles dropping our accuracy:
Release Notes: These were cluttering our retrieval pipeline with outdated details that didn’t help answer current questions.
Incident Reports: Useful during active issues, but once resolved, they became noise and were still being retrieved.
Product Launches: Exciting when first published, but their relevance faded as our products evolved.
We went ahead and filtered out these article types, and our retrieval accuracy jumped from 62% to 91%.
No retraining, no major overhauls - just better content hygiene and targeted filtering.
Unlike most of the stuff we do at Stylo, this wasn’t just about making our AI smarter. It was about putting our money where our mouth is.
Want to see how your knowledge base scores and what improvements you can make? Get in touch to run a Stylo Scorecard evaluation.